Revolutionizing Object Detection with Advanced Image Labeling Tools
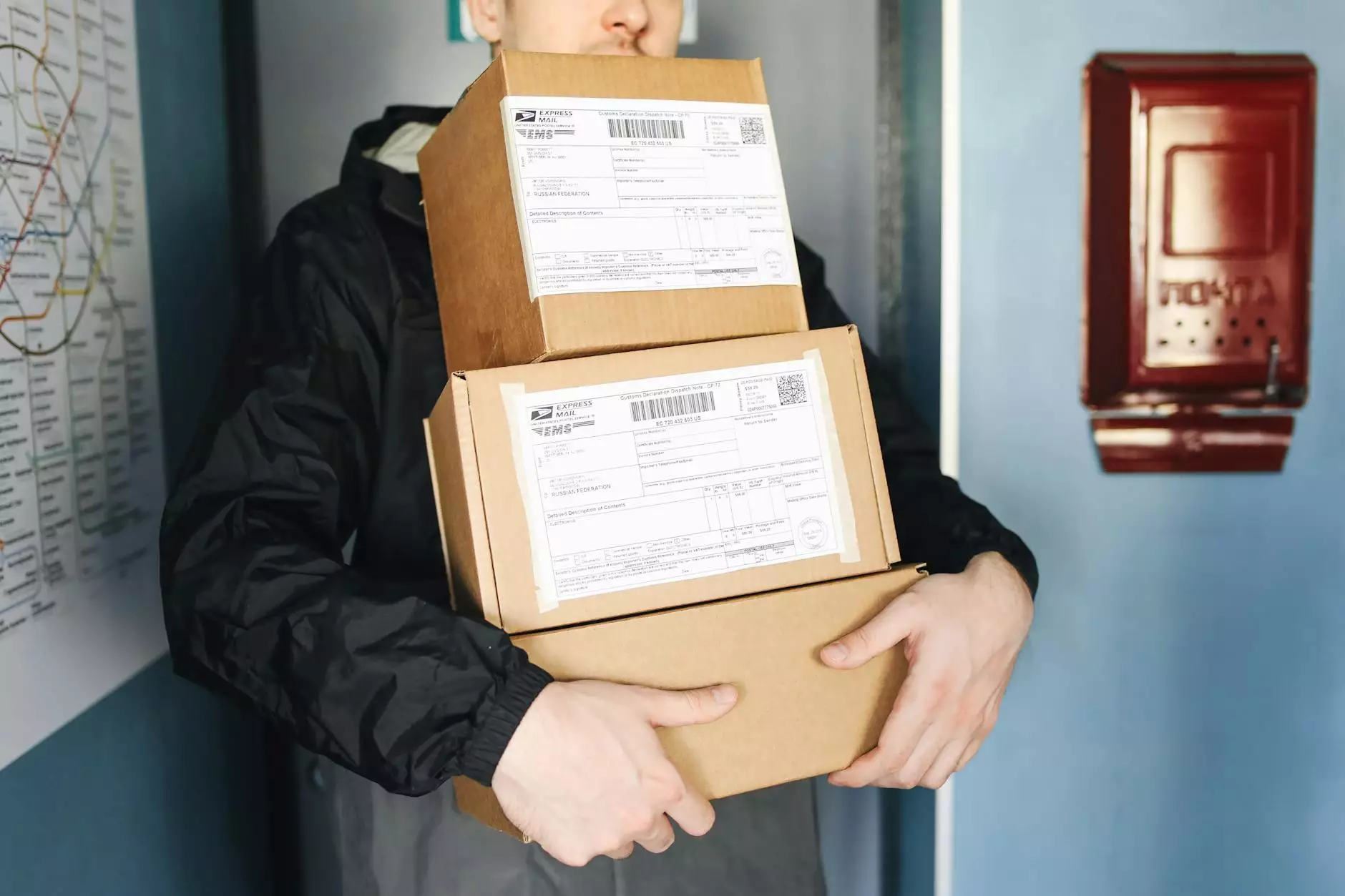
The evolution of artificial intelligence (AI) and machine learning (ML) has brought remarkable advancements in various fields. One critical aspect fueling this progress is data annotation, particularly through the use of image labeling tools for object detection. These tools play a pivotal role in training algorithms to recognize and interpret images effectively. In this article, we will explore the features, benefits, and best practices of using image labeling tools, specifically designed for object detection.
Understanding Image Labeling and Its Importance
Data annotation is the process of tagging or labeling data to train AI models. In the context of object detection, image labeling involves identifying and labeling objects within images. This practice is vital because:
- Training AI Models: Labeled images are essential for teaching AI systems to recognize objects in real-world applications.
- Improving Accuracy: The precision of the model heavily relies on the quality of labeled data.
- Reducing Bias: Well-annotated images help reduce biases in AI algorithms, leading to fairer outcomes.
What Makes a Good Image Labeling Tool for Object Detection?
A robust image labeling tool for object detection should encompass various features to enhance the user experience and improve annotation efficiency. Here are some crucial features to consider:
- User-Friendly Interface: A clear and intuitive interface allows annotators to work efficiently without extensive training.
- Multiple Annotation Styles: Support for bounding boxes, polygons, and point annotations to cater to various object shapes and sizes.
- Collaboration Capabilities: Enables teams to work together, share progress, and manage projects efficiently.
- Integration with ML Frameworks: The ability to export labeled data in formats compatible with popular machine learning frameworks.
- Quality Control Features: Mechanisms for reviewing and verifying annotations to ensure high-quality data.
The Process of Image Labeling for Object Detection
Understanding the workflow of using an image labeling tool for object detection is essential to optimize the data annotation process. The typical steps are as follows:
Step 1: Data Preparation
Before diving into the labeling process, it’s crucial to prepare your dataset. This includes:
- Gathering images relevant to your object detection project.
- Ensuring the dataset is diverse to cover various scenarios that the AI might encounter.
- Organizing the images systematically for easy access during the annotation phase.
Step 2: Choosing the Right Tool
Selecting an appropriate image labeling tool tailored for object detection is paramount. Consider tools that offer the features discussed earlier, along with support and community resources.
Step 3: Annotation Process
Using the tool, annotators will:
- Open images in the labeling software.
- Select the type of annotation (bounding boxes, polygons, etc.) for each object in the image.
- Label the objects accurately, ensuring comprehensive annotation for effective model training.
Step 4: Review and Quality Control
After annotation, it’s crucial to review the labeled data for quality assurance. Implement a review process that includes:
- Peer reviews among annotators.
- Using quality control metrics to evaluate consistency and accuracy.
- Final validation to ensure that the labeled data meets project specifications.
Benefits of Using an Image Labeling Tool for Object Detection
The implementation of a high-quality image labeling tool can bring numerous benefits to businesses:
1. Enhanced Productivity
With a streamlined interface and efficient annotation features, teams can label images faster, improving overall productivity.
2. Increased Data Quality
Using tools with built-in quality control mechanisms ensures that the labeled data is accurate and reliable, which is crucial for training effective AI models.
3. Cost-Effectiveness
Automating aspects of the labeling process can significantly reduce labor costs and time investment. This efficiency translates into savings for organizations.
4. Scalability
As projects grow, having a scalable image labeling infrastructure allows businesses to manage larger datasets without a hitch.
Key Labs: Your Go-To Solution for Image Labeling
At Key Labs, we understand the importance of quality data annotation. Our Data Annotation Tool is specifically designed to meet the needs of modern AI projects. Here are some features of our platform:
Comprehensive Annotation Options
We offer a variety of annotation styles suited for different use cases, ensuring that your project's unique requirements are met with precision.
Collaborative Environment
Our platform supports team collaboration, allowing multiple annotators to work simultaneously while monitoring progress in real time.
Integration Capabilities
Key Labs' annotation tool seamlessly integrates with popular machine learning frameworks, enabling easy export of labeled datasets.
Dedicated Support
We pride ourselves on providing excellent customer support, guiding you every step of the way in your data annotation journey.
Conclusion: Embrace the Power of Image Labeling for Object Detection
In today's data-driven world, leveraging an image labeling tool for object detection is not merely beneficial; it is essential for businesses aiming to harness the full potential of AI. By investing in quality data annotation platforms like those offered by Key Labs, companies can significantly enhance their machine learning models, drive innovation, and improve decision-making processes. Explore how our services can transform your data annotation experience today!